The lab was quiet, but not because the team had gone home. They were still there—tired, hopeful, half-running on instinct and half on caffeine—watching a simulation finish on a dusty monitor. One click, one result, one step closer to something that might finally work.
This is the rhythm inside a lot of R&D-heavy startups. Every day is a sprint toward a breakthrough. The budget doesn’t stretch, the hours don’t sleep, and there’s always a competitor somewhere, chasing the same solution with the same pressure. It’s not that these teams lack talent or ideas. It’s that research burns time. And time is the one thing they never have enough of.
So how do they stay in the race?
Not by throwing more people at the problem. Not by hoping for a lucky break. The smartest ones are building smarter labs—where AI isn’t a headline, it’s a helping hand.
When guesswork costs too much
A few years ago, a materials science startup spent nearly six months testing a new polymer blend. It looked promising on paper. It even passed the first few stress tests. But one variable—temperature sensitivity—kept skewing results just enough to throw everything off. They didn’t catch it until after the fifth round of expensive trial runs.
That mistake didn’t just cost them money. It cost them momentum. And in R&D, momentum is half the battle.
Guesswork isn’t always obvious. It hides in optimistic timelines, in untested assumptions, in data no one had time to fully clean. It’s the silent tax startups pay when they’re forced to choose speed over certainty.
That’s where the smarter teams started making a shift. Instead of trying to predict everything themselves, they brought in a different kind of partner—one that never tires, never forgets, and can spot patterns faster than any intern or overworked analyst.
It wasn’t about replacing their process. It was about removing the noise.
AI as the lab assistant that never sleeps
Jana, a biotech founder in her late twenties, used to spend nights flipping through dense academic journals, trying to spot patterns across studies that might validate her team’s direction. Now she runs machine learning models while she sleeps—feeding in molecular datasets, letting the algorithm surface combinations worth testing.
Her team still debates results. Still runs physical experiments. But they don’t start from scratch anymore. They start smarter.
AI in these labs isn’t a robot in a white coat. It’s a quiet partner—one that crunches what humans can’t, flags outliers, connects the dots no one saw coming. It doesn’t ask for lunch breaks. It doesn’t need weekends. And it doesn’t care how many variables you throw at it.
It’s not flashy. It’s functional. The edge isn’t in the code—it’s in how these teams are using it to stretch their brainpower further without burning out.
From hunches to hypotheses, faster
Most research teams are no strangers to gut instinct. A theory takes shape in someone’s head, and then it’s a matter of weeks—sometimes months—before they can test if it holds. That lag time eats away at budgets, morale, and often, the idea itself.
Startups using AI have trimmed that lag to a fraction.
Take a food science company working on plant-based proteins. Instead of testing each formulation manually, they trained an AI model on hundreds of variables—textures, temperatures, binding agents. What used to take a month to narrow down now happens in an afternoon. They still test in the lab. But they don’t waste time on dead ends.
It’s not about speed for the sake of speed. It’s about momentum. AI helps teams move from a “what if” to “here’s what we should try next” without stalling between steps.
The result? Fewer hunches. More informed hypotheses. And a lot less guesswork buried in spreadsheets.
Data as a living teammate
In most labs, data lives in folders—buried under filenames like final_final_results_v2. But in the smarter ones, it’s more like a teammate. One that listens, responds, and nudges the team when something’s off.
A robotics startup working on precision agriculture started treating their sensor data like feedback from the field. If moisture levels dropped even slightly outside the expected range, their system didn’t just log it. It adjusted the next irrigation cycle in real time—and sent the team a heads-up. What used to be post-analysis became mid-process correction.
That’s what shifts when AI gets involved. The lab isn’t just collecting data. It’s conversing with it.
Researchers still call the shots. But now, their tools don’t just measure—they interpret. And that kind of feedback loop changes how fast teams can move from observing a problem to solving it.
Small teams, bigger swings
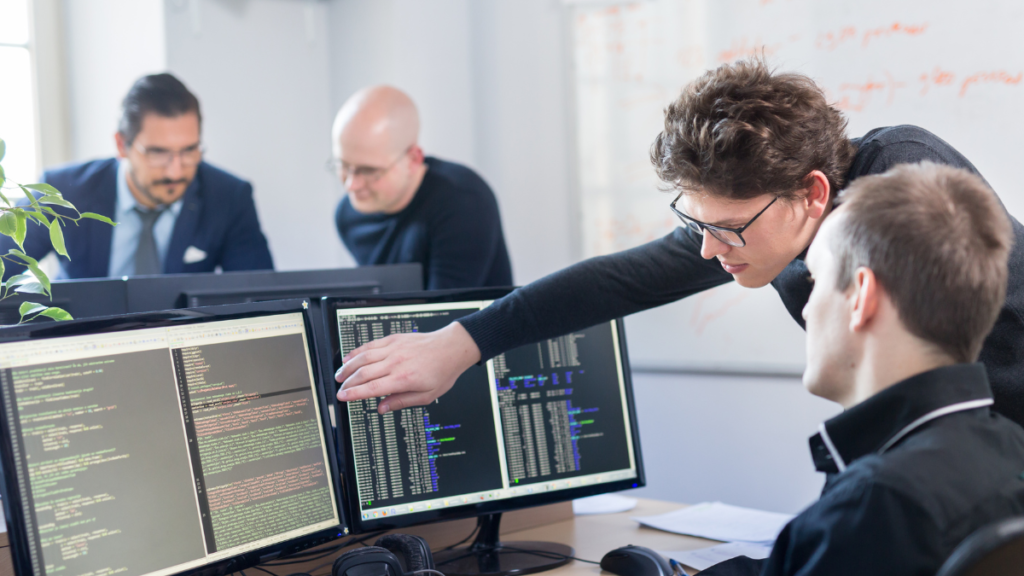
There’s a reason most deep tech breakthroughs used to come from well-funded labs with sprawling teams and massive infrastructure. Doing serious R&D used to mean hiring specialists for every sliver of the process.
Now? A four-person team can build a materials pipeline that would’ve taken twenty just a decade ago.
A startup in nanotech recently used AI to simulate hundreds of material combinations without physically producing a single one. They didn’t have a massive team—they had two engineers, a domain expert, and an AI specialist running models overnight. Within a month, they landed on a viable prototype that usually would’ve taken them a year.
It’s not about replacing people. It’s about stretching what a small, focused team can pull off when they’re not buried under the grunt work. AI doesn’t hand them the answers—it gives them the room to ask better questions and take bolder swings.
Pitfalls and growing pains
Not every AI-powered lab story ends in a win. Some end with corrupted data, mismatched models, or insights that look good—until they crumble under real-world pressure.
One early-stage medtech startup automated too much, too fast. Their AI flagged a compound as a breakthrough candidate. It passed initial digital simulations. But no one double-checked the source data. Weeks later, they realized the model had been trained on an incomplete dataset. The real-world version? Useless. Expensive. Set them back three months.
AI can accelerate. It can also amplify mistakes.
Startups that treat these tools like crystal balls often learn the hard way that AI isn’t magic—it’s math. And math is only as good as the data it’s built on.
That’s the line successful teams walk: trusting the system, but never blindly. Using AI to guide, not replace, the scientific process. The pain points are real. But so is the payoff—if you know where the boundaries are.
The startups writing their own playbooks
There’s no instruction manual for what these teams are doing. No pre-packaged solution that fits neatly into a lab running on tight margins and tighter timelines.
So they build their own.
A synthetic biology startup couldn’t afford enterprise software to connect its testing pipeline. So the lead engineer stitched together open-source tools, custom scripts, and a cheap AI model to automate sample tracking and predict error rates. It wasn’t pretty—but it worked. And it saved them thousands.
That’s the reality in these labs. The edge isn’t found in expensive platforms—it’s in creative workarounds, quick pivots, and duct-tape solutions that get better every week. These startups aren’t waiting for best practices. They’re writing them—one API call, one notebook entry, one model tweak at a time.
What they lack in polish, they make up for in grit.
The edge isn’t AI. It’s how these teams are using it
The real advantage isn’t in having access to AI. It’s in knowing what to do with it—where to point it, when to trust it, and when to question it. The R&D startups making waves aren’t the ones chasing trends. They’re the ones tuning tools to fit their weird, messy, brilliant process.
You won’t find glossy dashboards or perfectly labeled datasets in most of their labs. What you’ll find instead are late nights, half-broken prototypes, and a quiet hum from a machine crunching data in the corner. It’s not glamorous. It’s just work. Smart, risky, stubborn work.
And maybe that’s the best definition of an edge: not something flashy, but something that keeps you moving when most would stall.